In today’s data-driven world we have access to more information than ever before. At Coppett Hill, we use data to help our clients make strategic and operational go-to-market decisions, to unlock growth. But as the saying goes, “garbage in, garbage out” (GIGO). Without good quality information, even the most sophisticated analysis will deliver unreliable outcomes. This post explores why clean, accurate data is the foundation of successful decision-making, and how poor data quality can lead to misguided strategies, wasted efforts and potentially costly mistakes.
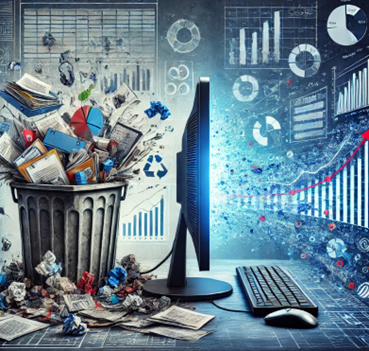
Understanding the GIGO Principle
GIGO is a simple yet powerful principle – the quality of input data directly impacts the value of the output. If your input data is flawed — whether due to inaccuracies, omissions, or biases — the analysis will be skewed, and any decisions made on that basis are likely to be misguided. For businesses, this can mean missed opportunities and misallocated resources.
To counter this, I’ve developed a habit of always asking where the data/information comes from, and how it was collected – a habit that I suspect many other consultants will recognise.
Consider a company trying to define its Ideal Client Profile (ICP). If the data used to understand existing customer lifetime value is incomplete or outdated, the analysis will provide a distorted view of customer ‘likeability’. Decisions based on these insights could result in poorly targeted marketing, unprofitable product launches, and misalignment with customer needs.
Why clean, high-quality data is essential – avoiding ‘garbage in, garbage out’
Accuracy in decision-making: High-quality data provides the foundation for strategic decision-making. When data is clean, consistent, and relevant, it allows businesses to identify patterns, make forecasts, and evaluate risk effectively. Low-quality data, on the other hand, introduces uncertainty, forcing decision-makers to rely on assumptions or ‘gut-feel’ that could result in sub-optimal strategy.
Efficiency in resource allocation: Decision-makers rely on data to allocate resources effectively, whether that’s budget, staff time, or technology investment. With accurate data, businesses can allocate resources confidently. Conversely, poor-quality data may direct resources toward less impactful areas, leading to wasted budgets and missed opportunities.
The hidden costs of poor data quality
While it’s easy to see the immediate costs of bad data, such as wasted time and missed revenue opportunities, there are also hidden costs that can be even more damaging in the long run.
Increased operational costs: Poor data often requires rework. When errors are discovered, time and resources are allocated to correct them. How often do simple reporting requests result in hours (or even days!) of manipulation to give the desired answer? The time spent cleaning data or backtracking on misguided decisions could be better invested in productive activities if quality data were used from the outset.
Decreased team productivity: When analysts and team members spend their time cleaning and validating data rather than conducting meaningful analysis, productivity suffers. Poor data quality increases frustration and decreases morale, as team members are constantly fixing preventable issues. I experienced this in a previous role, where the monthly board reports took 2-3 working days to create due to data silos and manual extraction – when reporting becomes a major undertaking itself, it can cause significant frustration within the team.
Erosion of Trust: If decision-makers lose confidence in the data provided, they are less likely to trust future analysis. This trust is difficult to regain and can impact the effectiveness of future analysis. Teams may become more hesitant to rely on data insights, leading to a “gut-feel” approach that is inherently riskier.
Implementing good data practices to avoid GIGO
The good news is that organisations can avoid the GIGO pitfall by implementing robust data hygiene practices. We have seen these methods work firsthand for many of our clients, within operational finance systems, sales & marketing systems, and third-party media platforms:
Defining clear data standards & definitions: Different departments may collect and format data differently, leading to inconsistencies. Define clear standards for data collection, entry, and processing across the organisation to ensure that everyone follows the same guidelines. Use a ‘data dictionary’ to define each dimension and metric consistently. If these change due to a shift in business strategy or organisational design, ensure that changes are backdated. Data within a business is often human generated – think Sales team members filling in opportunity details in a CRM system, or customers filling in feedback forms. With human intervention comes an even greater need for review and classification, to avoid inconsistencies which will impact your analysis.
Data cleansing and validation: Establish processes to routinely cleanse and validate data, identifying and correcting any errors before analysis begins. Automation tools or data validation can help by flagging duplicates, missing values, or outliers, but human oversight is also essential for ensuring contextual relevance. For example, when we build Customer Data Platforms we will often inspect many individual customer journeys to ensure that they are (a) logically complete and (b) correctly segmented.
Investing in high quality data sources: High quality data often requires a financial investment. Whether it’s subscribing to reputable data sources or investing in data-gathering technologies, this upfront cost will likely save significant time and resources by providing reliable input for analysis.
Regular data audits: Schedule regular audits to assess data quality and make improvements where needed. Audits help catch errors early and give stakeholders confidence in the data’s reliability. Additionally, regular reviews help identify evolving needs that may require adjustments to data collection processes. One tip we encourage is building data hygiene reports into your regular sales and marketing reporting (i.e. a standalone data quality dashboard for CRM data entry) to incentivise compliance among the sales team.
Training and development: Equip team members with the skills and knowledge to recognise data quality issues. When your team understand the importance of high-quality data and know how to handle data correctly, the organisation is better positioned to maintain high standards over time.
Prioritise quality data for quality outcomes
The “garbage in, garbage out” principle is a reminder that even the best analytical methods can’t overcome the limitations of poor-quality data. By investing in quality data practices, businesses can make better decisions and more effectively allocate resources. As the reliance on data continues to grow, the importance of quality inputs cannot be overstated. When quality data is at the heart of analysis, businesses are not only better equipped to avoid costly mistakes but are also positioned to capitalise on the full potential of their data.
In the end, making good decisions starts with good information. By ensuring your data is clean, relevant, and reliable, you’ll have the insights needed to make strategic choices that drive sustainable growth and success.
If you’d like to discuss how to avoid ‘garbage in, garbage out’ within your Sales and Marketing systems, to ensure better go-to-market decision-making, please contact us.
All views expressed in this post are the author's own and should not be relied upon for any reason. Clearly.